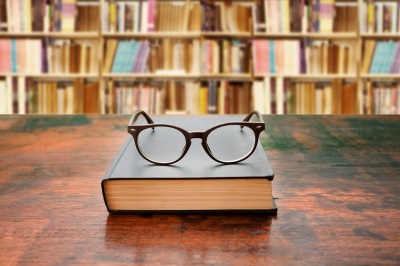
August 3 2010
This three part blog has been reviewing the logic and use of predictive modeling in admissions work. In my previous posts, I provided an introduction and overview to predictive modeling, selection of variables, and what the output of predictive modeling tells the end user. In this final posting, I am going to discuss the use of additional variables, not provided by the inquiry, in predictive modeling. As I indicated in the second blog of this series, inquiry to enrollment predictive models use variables that admission counselors routinely collect at the point the inquiry first expresses interest in the institution. Typically these variables include source or referral code, major, contact information, and demographic information about the inquiry. While these data points are useful, it is beneficial to supplement these variables with additional pieces of information. One possible avenue is to use information about the inquiries neighborhood. An inquiry’s zip code can be used to gather information about the neighborhood in which the inquiry resides. A variety of vendors provide information about zip codes that can be used in predictive models. For instance, at Credo we routinely use information about the education level of a zip code, median family income, median home value, level of diversity, and population density in predictive modeling. Conceptual support for using information about an inquiry’s zip code is found in educational research. Generally speaking, homogeneity is found in clusters, and zip code is a type of cluster. By that I mean, in general, that people tend to live (or cluster) with individuals that are similar to themselves. Using estimates about the qualities and characteristics of zip codes allows for a more accurate understanding of an inquiry pool. For instance, including qualities and characteristics of a zip code in a predictive model allows you to understand if inquiries that come from racially or ethnically diverse neighborhoods are more likely to enroll at your institution. It also allows you to draw conclusions about the socioeconomic diversity of your inquiry pool and the influence that socioeconomics have on an inquiry’s likelihood to enroll. The inclusion of data about a zip code in a predictive model is not without its complications. The use of these data points required appropriate methodological treatment, primarily through the use of multilevel modeling. Multilevel modeling accounts for the nested data structure that is inherent with the addition of data about an inquiry’s zip code, where inquiries are nested in zip codes. Predictive modeling can be a complicated process that requires some advanced training in statistics; however, it is within the grasp of every admission director and counselor to understand the output of an inquiry to enroll predictive model, and use that information to influence how they build their class.
Recent Posts
- Richard Dunsworth, J.D., To Receive 12th Annual Courageous Leadership Award December 16 2024
- Meet the 2024 Credo Values Award Recipients August 27 2024
- What is Executive Coaching, Really? June 25 2024
- Credo & The Constructive Dialogue Institute Announce A New Partnership For Higher Education Leadership Development March 7 2024
- Celebrating Women And Gender-Diverse Learners & Leaders February 28 2024
Categories
- Strategy
- Leadership
- Student Success
- Student Success & Retention
- Enrollment
- Pivot
- Strategic Planning
- Leadership Development
- News
- Strategic Enrollment
- Campus Planning and Architecture
- Moving the Needle
- Data
- Research
- Retention
- Campus Master Planning
- Enrollment & Financial Aid
- Academic Programming
- Campus Planning
- Thriving
- Admitted Student Research
- Architecture
- Finance
- Advising
- Admissions